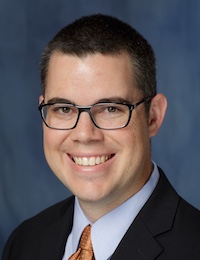
In Conversation with...Patrick Tighe about Artificial Intelligence
Editor's note: Patrick Tighe, MD, MS, is a practicing anesthesiologist at University of Florida Health (UF Health) and the executive director of UF Health’s Quality and Patient Safety Initiative. We spoke to him about the current and potential impacts of artificial intelligence (AI) on patient safety as well as challenges to successful implementation.
Sarah Mossburg: Welcome, Dr. Tighe. Could you tell us about yourself and your current role?
Patrick Tighe: Hi! I’m Patrick, and I’m an anesthesiologist at the University of Florida. Clinically, I specialize in regional anesthesia and perioperative pain medicine. I’m currently the Executive Director of the Quality and Patient Safety Initiative in the College of Medicine at the University of Florida.
Sarah Mossburg: We’re here to talk to you today about artificial intelligence and machine learning applications in healthcare. How do you define artificial intelligence and machine learning?
Patrick Tighe: AI is short for artificial intelligence, but I think more conventionally these days, we talk about it as augmented intelligence—keeping human beings at the center as much as possible. AI encompasses a lot of different domains, but at the root of each of those domains is trying to teach machines how to solve problems like a human would, or potentially even better. Machine learning is a subset of AI, and it involves algorithms that can learn from experience. We give the algorithm training examples; it learns the patterns of those training examples and can then infer those patterns onto new cases that it’s never seen before.
Machine learning is probably one of the most common types of AI that has been investigated for use in healthcare. Over the past decade, whenever we’ve talked about doing AI, we’ve usually been talking about machine learning. That’s starting to change now with more applications of deep learning and large language models in healthcare, but I think that’s going to be the bulk of what you will see when encountering AI in the clinical space.
Sarah Mossburg: What do experts hope AI or machine learning will add to the current landscape of patient safety?
Patrick Tighe: One of the most exciting aspects of AI is the ability to access more data. Traditionally, to complete statistical analyses, we need to get data into a structured format. It had to be formatted like an Excel spreadsheet, with neat rows and columns, and carefully defined elements in each of those rows and columns. In healthcare, there are massive amounts of data from sources like clinical notes, imaging, and physiologic waveforms. There are data about what our patients are doing during their recovery from illness. For instance, how they move after surgery or how they complete a digital clock drawing test. These are really important details, but you can imagine the difficulties with squashing a chest x-ray or mammogram into a spreadsheet! With AI tools, we can capture this information, combine it with other data, and put it all together into a single model without spending weeks, months, or years on customized, per-project “feature engineering.” The flexibility to generalize this information into a common stream that is analyzable is really helpful. And this access to more data can help a multitude of modern issues in healthcare.
Another area that I am really excited about is continuous patient monitoring. Instead of monitoring once an hour or once every 15 minutes, we can monitor patients on a minute-to-minute or even second-to-second basis over time. For example, in an intensive care unit, patients who have delirium can wax and wane in their symptoms, and we may not be sampling their status enough. What if we could monitor continuously and much more accurately characterize their neurocognitive status? That’s a major advancement. Similarly, can we use AI to develop more useful alarms that are in the context of everything else happening with the patient, so that we don’t suffer from alarm fatigue.
In terms of improving access to care, hospitals are bursting at the seams across the United States right now, and it’s limiting access to healthcare and causing its own set of patient safety issues. AI can potentially allow us to use our existing healthcare resources more efficiently and look for opportunities to deliver safer, more effective care outside of the hospital. I think we can do that through better use of unstructured data, better understanding of patient flow and the likelihoods of patient outcomes, and better monitoring of patients and clinical care in nontraditional settings.
The final area that I’m interested in using AI for improving patient safety is in multi-objective optimization and reinforcement learning. Up to this point, a lot of our models for patient care only focused on one outcome at a time. But decision makers, patients, and clinicians are almost always balancing multiple considerations at the same time. For instance, a medication may relieve pain after surgery, but depending upon the medication, there may be a risk of respiratory depression, medication addiction or dependence, or kidney injury. But if there is a decrease in pain, there is a potential for improved ambulation and faster recovery from surgery, among other benefits. How do we balance all of these factors and put them together to optimize the care for a single person? Humans often do a good job of balancing these concerns, even concerns that our patients share with us but are not quantifiable. Balancing those becomes a very human activity and often difficult to quantify with contemporary AI methods. I think the move toward multi-objective optimizations will be helpful, but we’re not there yet. We still have some work to do.
Sarah Mossburg: Really interesting. You raised a lot of really interesting potential for the future. Let’s step back briefly and talk about the areas that AI has been used in healthcare historically. How have those uses intersected with patient safety?
Patrick Tighe: One of the most common uses of artificial intelligence in recent years has been predicting adverse outcomes using risk-scoring systems. For example, what is the chance that a patient will suffer a complication after surgery or is in the early stages of sepsis? Before AI, we had pretty good statistical models built on logistic regression, but we continue to see more and more complicated AI algorithms with richer and richer datasets that lead to better prognostic performance. The core presumption is that if we can predict an adverse outcome, we can change the direction of that outcome.
Another increasingly common use of artificial intelligence is in looking at radiology images to help manage findings. Computer algorithms can help pick up tiny signals on a chest x-ray that humans may have difficulty seeing after even after decades of training. AI can help put findings all together in terms of the clinical picture. There may be opportunities to use findings as a cognitive assistant for radiologists who are looking at imaging to complement their considerable skills and expertise. For instance, in a queue of dozens or even hundreds of images awaiting a radiologist’s review, an AI solution could help prioritize which studies should be reviewed first.
Sarah Mossburg: What has research shown about AI and its potential to improve patient safety?
Patrick Tighe: Strictly speaking, artificial intelligence itself may not be able to lead to demonstrable improvements in patient safety. Rather, it’s the actions that we take based on the patterns that emerge from AI-based analyses that can lead to these improvements.
There have been research findings to suggest that AI can detect certain events earlier, and that in some cases, this may lead to substantial improvements in outcomes when clinical teams act on those alerts. In some reports, AI solutions that address deterioration from sepsis and other conditions have been associated with significant reductions in mortality across multiple health systems.
In these programs, AI has been part of broader quality improvement (QI) initiatives that led to outcomes. It’s difficult to tease out the components of QI results that are directly attributable to AI. Several of the QI activities that involve AI that we’ve been a part of have helped us take a different look at the challenges we’re trying to address. In some cases, just taking that second look at a problem with this set of AI-fueled lenses leads us to change how we’re going to address it even before we got to an AI-based solution.
Sarah Mossburg: I’m thinking back to the point you made earlier: that you can predict who’s at risk, but that doesn’t necessarily change the outcome, and that the actions that we take are a critical part of that as well. Can you elaborate on that?
Patrick Tighe: For instance, let’s say we have an algorithm that predicts the likelihood of a patient developing sepsis after surgery. We can look at three potential scenarios.
First, we have a patient who is hypotensive, tachycardic with fever, and positive blood cultures immediately. The clinician might already be moving the patient to the ICU and may have started antibiotics. The AI algorithm then says, “We think your patient may have sepsis.” In that case, there is not a lot of clinical value from that estimate. If you look at the performance of the AI algorithm though, it predicted the patient was septic, and so the algorithm looks great!
In another scenario, we have a healthy 22-year-old patient presenting with normal vital signs who remains highly functional after surgery, but the AI is saying the patient is septic. The clinician is looking at this patient thinking, “I don’t think so….” This is the exact patient that a lot of us want AI to work on, where we’re completely blindsided by the case. The clinician may be in a bind about whether to admit the patient to the ICU. If the patient does develop sepsis eight or 12 hours later, and then we start treatment, then the AI was right, it predicted something that nobody else thought was happening. Yet the clinical impact was not great, because the patient still went on to develop sepsis, because we didn’t believe it. That’s another example where the AI performance is separate from the clinical impact.
We can look at a third case with another perfectly healthy 22-year-old patient and the AI algorithm suspects sepsis, despite close to normal vital signs and a reassuring overall clinical picture. Yet in this case, the clinician says “I believe this AI algorithm. I’m going to initiate treatment right away.” And lo and behold, the patient never really gets much sicker and never met the criteria for sepsis! Afterwards, people will say that the AI algorithm was wrong, yet it actually conveyed the clinical impact that I think we want to see. We call this confounding by medical intervention, and it further highlights the potential for clinical impact to differ from the usual metrics of model performance.
So, which of the three cases is the best scenario there? What do you do when you disagree with an artificial intelligence algorithm? How do you know you’re doing better than what you might have done otherwise? Who assumes the liability for agreeing, or disagreeing, with an AI recommendation? I think these are important question that we’re going to start running into, but it also showcases how AI can link to those processes and decisions that lead to improvements in unexpected ways for patient care.
It’s important to recognize that a lot of our algorithms at this stage are incorporating the prediction of one outcome, but a patient brings with them an understanding of multiple outcomes, as well as which of those outcomes are most important to them. Most of our solutions don’t really consider that, so we’re back to augmenting intelligence rather than replacing a clinician’s judgment.
Sarah Mossburg: Could you give us an overview of some of the work that you’ve done related to AI and quality improvement and how that can potentially improve patient safety?
Patrick Tighe: Yes, I’ll start with a vignette of one of our “learning occasions” that was quite eye-opening. One of the first projects we worked on was predicting pain after surgery. The hope was that if you knew that a patient was going to have severe pain after surgery, the algorithm would help predict if you should intervene, for example, with multimodal analgesics more aggressively to prevent that pain. We assembled a large number of features about our patients, and we put them into an algorithm that would predict the likelihood of severe pain after surgery. Our model performance was okay and contemporary for the time. I remember presenting the algorithm to my clinical mentor and my mentor asked what I thought should be done for a patient that is at risk of severe pain after surgery. I said I think you should do something like recommending an epidural catheter to the patient, to which he responded that he was going to do that regardless! He then asked me if my model accounted for all the decisions that we were going to make based on what we thought was going to happen to the patient; in other words, the space between the preoperative features and the postoperative outcomes, the space that we had some agency to hopefully modify toward a better outcome. He was absolutely right. It really humbled us and made us realize that just being able to predict an outcome is not the same as effecting an improvement of that outcome. It also led us to look into different AI methods that could help with this puzzle, such as reinforcement learning.
Another area in the perioperative space that we’ve worked on with AI is understanding how well a patient’s brain is functioning before surgery and what that baseline brain functioning portends for them having a neurocognitive disorder after surgery. We worked with Dr. Cate Price and a number of others on an AI analysis of the digital clock drawing test.1 In just a couple minutes, we can get hundreds of thousands of pieces of information about how a patient’s brain is working during the clock drawing task. We can use that data to help predict outcomes for those patients, such as if the brain may have some difficulty recovering from the stress of surgery. We still have a lot to learn, but it’s a game changer in terms of the information we have access to and what we can do with it.
I have colleagues that are using video recordings of patients to look at facial expressions for pain, for delirium assessment, for mobility. You can see how that can lead to safety. These cameras can do this all of the time. This idea that you can have a watchtower that’s keeping an eye on the patient as a supplement to the existing monitoring modalities we have is really helpful. We have a shortage of healthcare professionals. Yet we know that some of these conditions wax and wane. If you’re only checking in on this patient once every 15 minutes, or even once every 5 minutes, you can still miss short episodes of change here and there. It’s a really exciting opportunity to not just keep an eye on the patient, but quantify certain aspects of their movement, and you can put it all together into a more holistic picture of how they’re recovering. This may also allow us to help take care of more patients with the same amount of staff.
In other areas, we’re developing AI solutions to facilitate processing of geospatial information to help us understand how we can better link patients to community resources to address social determinants of health, to better schedule hospital patients for follow-up clinic appointments, and to better understand health systems as complex dynamic systems to improve patient access to our hospitals and clinics.
Sarah Mossburg: We’ve been talking a lot about potential benefits of AI, and it seems like there are many. What are some risks of AI implementation to patient safety that we should be cognizant of?
Patrick Tighe: The first thing to note is that only about 21% of Americans are excited about artificial intelligence, the rest are neutral or concerned about AI and its growth.2 Some people are worried that AI is going to take over the world in very dramatic fashion. I suspect there are also more subtle issues of trust in AI present, even among enthusiasts. It may recommend movies to me, or things to buy at the grocery store, but it doesn’t always get that right, and those are low-stakes issues. It’s fine if it suggests the wrong movie for me, but what if it gets it wrong when it comes to my healthcare? Suddenly I have higher standards for it.
Closely related to trust is the idea of interpretability or “the black box phenomenon.” Numbers go in, recommendations come out, and we’re not exactly sure what happened in the middle. I think many times as humans, we’re okay with those predictions as long as we agree with them. But when they diverge from what we expect, it becomes more problematic. We talked about some case studies earlier related to that, and a lack of interpretability hurts the trust we have in these algorithms. Those disagreements will inevitably happen with AI systems.
Another big challenge I see, especially with the more advanced methods like large language models, is called alignment. What do we want to get and what are we asking these algorithms to do? It’s very hard for me to ask a system to perform something when I don’t know what I want out of that system. A lot of us have played around with ChatGPT and other large language models and have been shocked at how helpful and accurate they can be. But I think in the next few years we will begin to see concerns of, “How do we make sure the responses comport with our internal set of values?” and “When is accordance necessary, or at least desirable?” We live in a pretty heterogeneous society, and the diversity of thought also conveys into the health setting. So how do we ensure that recommendations are aligning with patient values and what they are looking to get out of a recommendation system? And what are the ultimate goals of that decision support system?
Finally, another set of risks is this idea of handoffs during human-machine teaming. When does an AI system admit that it’s not confident and it wants or needs to hand off to a human? This was an issue in self-driving vehicles for a while: how does a car that’s going 60 miles an hour smoothly hand off to a driver that may have been disconnected from the driving process? We can imagine similar issues in healthcare where an algorithm is making good decisions and then runs into something it’s never seen before. That handoff back to humans is a fundamental concern for a lot of AI systems across industries.
Sarah Mossburg: Do you have any thoughts around how models can be validated and monitored to ensure high-quality results and patient safety in a real-world scenario?
Patrick Tighe: The traditional response is to create generalizable models that work across multiple sites. If it works really well at one site but not at other sites, the suggestion is that we have to change it so that it works equally across all sites. Another approach is to have a model for site A that works well at site A, and then we’re going to have a separate model for B that works well at B, because those are in two different cities, serving two different populations. By generalizing it too much, we’re possibly not paying attention to the particular needs of patients at these different sites.
I think we need to do sensitivity analysis about how these models work for segments of the population and how much trust can we put into a model for its estimate for that patient. I think demanding that of our models is very helpful and it promotes trust.
Another consideration is that many of us thought that we’d be able to create a model, launch it into practice, and walk away, but given various modes of model drift and confounding medical intervention biases, many models are going to require continuous maintenance. We’re going to have to update them on a regular basis, reevaluate their performance, monitor them for further drift, see how they’re changing. We often think of drift in the sense of new types of patients entering into the system, but drift can also result from changes to diagnostics, treatments, and other aspects of healthcare delivery, including other AI models! We also need to make sure that the outcomes of interest are stably defined and haven’t changed. Health systems are going to need infrastructures that can manage this over time. Perhaps this isn’t terribly dissimilar from hospital pharmacies. It’s rare that we introduce a new medicine, and that’s it. There are new medicines, changing indications and contraindications, updated safety information about medicines. There are pharmacy and therapeutics committees at hospitals continually looking at this information, monitoring antibiotic resistance rates, for instance. I think you’re going to see a need for similar philosophies for AI decision support tools. (And, I might suggest, just like we’re all pretty grateful to work with great pharmacists in this regard, I think we’ll also be really grateful to work with great clinical AI engineers!)
Sarah Mossburg: In terms of strategies or practices for organizations that are integrating AI, would you suggest looking at current strategies and practices for implementing new technologies, medications, procedures, and aligning with AI?
Patrick Tighe: Yes, and that’s a continuous, never-ending process. There are also more and more tools to make that monitoring more effective and more efficient. It’s going to require a multidisciplinary team with some transdisciplinary training as well among them, because no one person has the ability to maintain expertise in all these areas.
Sarah Mossburg: What aspects of healthcare would you say cannot be replaced with AI? What role are clinicians playing in an AI-augmented future?
Patrick Tighe: Compassionate care requires a person to deliver this care. There are machines that can mount empathetic responses, but there’s something about being there, having a shared experience. We all want the grand slam solution for making patients better, but there are also so, so many small things that clinicians do for our patients on a minute-to-minute basis, that for many patients eclipses the limitations of what we can do medically. For example, holding a patient’s hand, supporting them, and coaching them through their first steps as they get out of bed. It’s the team of folks that come together to help a birthing parent through childbirth and afterward. The computer can help a little bit and can maybe dispense good advice, but that’s far from the whole of medicine. It’s not just what we did, it’s the how we care for somebody.
From a safety perspective, especially in Safety II, humans also help “mind the gaps” in complex systems. AI systems are trained on training data; in other words, on patterns they’ve seen before, on known failure modes. They can offer robust solutions, but robust solutions can become brittle toward new vectors of failure. Humans offer resiliency against the unforeseen.
One of my big hopes for augmented intelligence in healthcare is that it helps alleviate some of the burnout and inefficiencies in healthcare, which will let people get back to taking care of people. That is the quintessential reason that all of us went into healthcare. Maybe AI can get us back to doing that and get us energized to do it well for the sicker and sicker patients we all care for. That’s something AI can’t replace. It can help us, but it’s going to help us by letting us do it, not by doing it for us.
Sarah Mossburg: Is there anything that we didn’t discuss today that you’d like to bring up?
Patrick Tighe: We didn’t talk much about large language models and where that’s headed in the future. Historically, AI has been seen as this domain of computer science and computer scientists who could code. We spent a lot of time on math and different types of algorithms. With large language models now, I can tell an agent what I want to do, and it will create the computer code for me. Instead of our attention being focused on algorithm development and computer coding, there’s an opportunity to shift toward how we want this system to benefit our patients, to benefit our health systems. It is no longer going to be a strictly technical exercise. Implementing AI is going to require more creativity, and large language models will remove a lot of barriers to who can do this. Algorithms will be able to be created by someone without a four-year computer science degree, an eight-week coding boot camp, or even a two-week introduction to coding. The challenge will be how creative can we be to leverage these tools effectively and bring about the changes that we want to see for and with our patients? That’s going to democratize this process heavily. We won’t pass this off to the technical experts, it going to be in our own hands.
I know there’s a lot of concerns about AI replacing jobs, but I would instead focus on all those tasks that are on the wish list. The “could have, should have, would have, but we would never get around to.” Such as, “What if we could track all of these outcomes two or three or five years out for all of our patients, all of the time?” Suddenly that changes how we’re looking at the well-being of our patients. The job didn’t go away. It actually probably led to more jobs and more opportunities, all of which allowed us to take better care of more and more patients, which is what we’re going to need to do anyway.
Sarah Mossburg: That’s really interesting. Thank you for chatting with us today, Dr. Tighe.
1 Bandyopadhyay S, Wittmayer J, Libon DJ, et al. Explainable semi-supervised deep learning shows that dementia is associated with small, avocado-shaped clocks with irregularly placed hands. Sci Rep. 2023;13(1):7384. doi.org/10.1038/s41598-023-34518-9
2 Artificial Intelligence Policy Institute. Poll shows overwhelming concern about risks from AI as new institute launches to understand public opinion and advocate for responsible AI policies. Accessed February 26, 2024. https://theaipi.org/poll-shows-overwhelming-concern-about-risks-from-ai-as-new-institute-launches-to-understand-public-opinion-and-advocate-for-responsible-ai-policies/